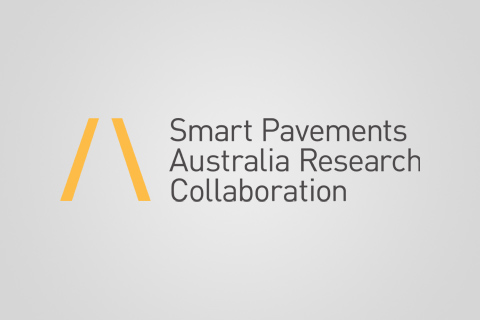
Project 3.5 (New Project Number 18.1, 2022)
Background
The problem of maintaining roads to an acceptable level with the resources available is a complex decision theoretic scheduling optimization problem. It involves the modelling of pavement deterioration, dealing with seasonal variations, and ensuing that urgent upgrades are scheduled in time with the minimum amount of disruption. In this project, we plan to use machine learning methods and big data analytics to model the seasonal variation of roughness and the deterioration of pavements.
One of the challenges in this project is to understand and select the appropriate data that provide useful information regarding climate, traffic maintenance, condition and geology. These data are collected from various sources and are not in structured formats. Big data integration and validation models are adopting to process the available data and get them ready for further knowledge discovery. Using these models, we will then develop an efficient method for sampling pavement condition data.
Project Objectives
1. To undertake a thorough review of existing methods for big data integration & validation, and modelling pavement deterioration.
2. To select the appropriate data sources collected for climate, traffic, maintenance, condition and geology as the materials for machine learning and big data analysis.
3. To integrate big data in various formats and structures from vast sources using big data pre-processing and validation methods.
4. To develop a predictive model for modelling pavement deterioration using machine learning.
5. To develop comparative classical statistical models and evaluate the relative performance.
- Dr Jackie Rong (LCI - Monash University)
- Dr Yihai Fang (Monash University)