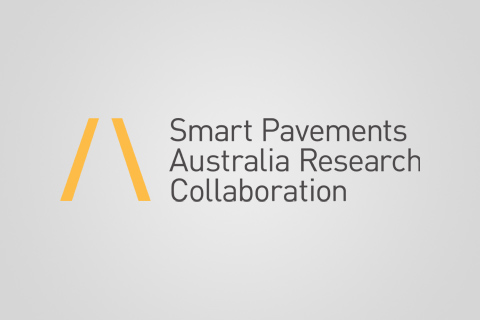
Project 3.3 (2019)
Background
In situ pavement density is critically important to flexible pavements because it is directly related to structural capacity and service life. Inappropriate density can cause premature pavement failures and subsequent extra cost in maintenance and rehabilitation. In current practice, density is commonly measured by using a nuclear density gauge, which however can only provide results at discrete testing locations. To overcome the limitations of the traditional method, new sensing techniques have been developed including thermal imaging, ground penetrating radar (GPR), and electromagnetic (EM) density gauge.
As the quality of newly constructed pavement depends on the quality of compaction during the construction of pavement, the progress of pavement density should be monitored after each roller pass until the desired density has been achieved. Although measuring pavement density using new sensing techniques has been achieved, these are generally applied only after the construction of pavement. In practice, the compaction pattern to achieve the desired density during construction is still established on the basis of experience and trial and error, which is time-consuming and fraught with risk. In this respect, intelligent compaction (IC) technology has been developed to monitor the field compaction of pavements in real time.
As modern vibratory rollers, IC is equipped with an integrated multiple sensor based measurement system, an on-board computer reporting system, GPS based mapping, and optional feedback control. The operator could then view the density formation in real time and adjust compaction parameters. It is therefore beneficial to develop a tool that can collect data continuously without contacting the pavement surface, and interpret the data in real time for quality control and quality assurance of pavement construction.
Project Objectives
1. To conduct a comprehensive literature review of remote sensing techniques e.g. LiDAR, GPR and GPS forpavement density measurement
2. To establish a database of sensor data and key parameters that are relevant to density measurement and prediction
3. To develop a model-free material density prediction approach using artificial intelligence
4. To design a system which integrates advanced sensors to monitor the compaction of pavement and predict pavement density in real-time
- Professor Jayantha Kodikara (LCI - Monash University)
- Professor Jeffrey Walker (Monash University)
- Dr Ye Lu (Monash University)
- Dr Yihai Fang (Monash University)